In the development of extended reality applications, our center focuses on two major themes: motion capture and training, and content co-creation and co-performance.
The forward-looking research goal is to develop AI technologies for 3D content generation, including using GPT architecture to achieve bidirectional generation of text and motion, and to recreate 3D scene objects, character models, actions, and expressions.
Additionally, we will explore the generation of extended reality content based on multiple sensory inputs (such as touch, emotion, and intention).
By capturing real-time information of real scenes/objects/characters (including images, audio, movement information, physiological signals, etc.), we will use AI and extended reality technologies to analyze and recreate 3D models of scene objects, character models/actions/expressions, and the sounds, touch, and smells of scene objects.
This will achieve various applications integrating virtual and real environments, such as remote social interaction, medical treatment, education, retail, entertainment, and tourism.
Finally, our center will integrate the above three forward-looking technologies to achieve vertical applications in sports technology and content co-creation, with the main focus on assisting athletes in improving their performance and innovating in artistic performances.
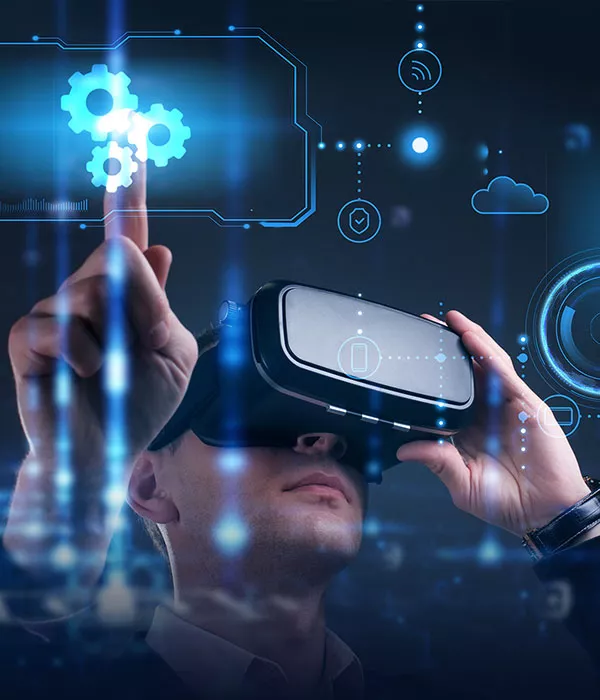
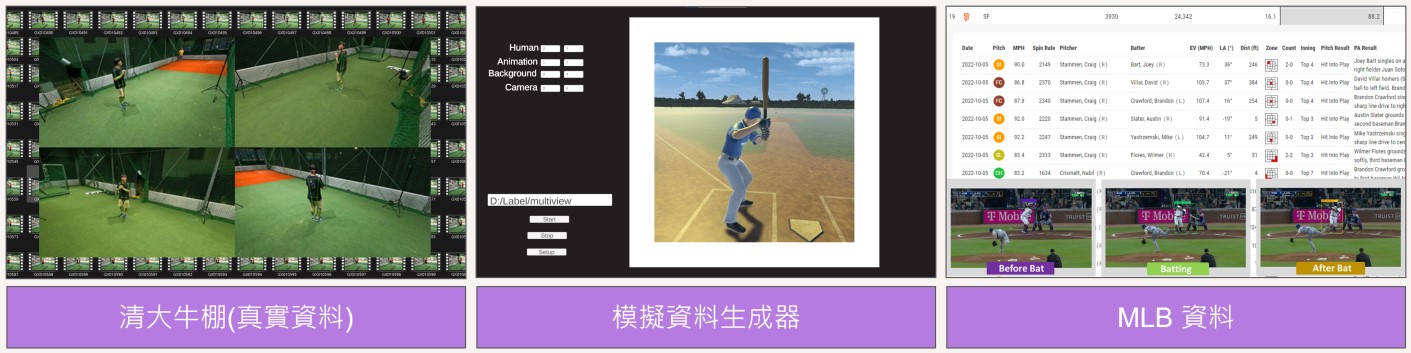
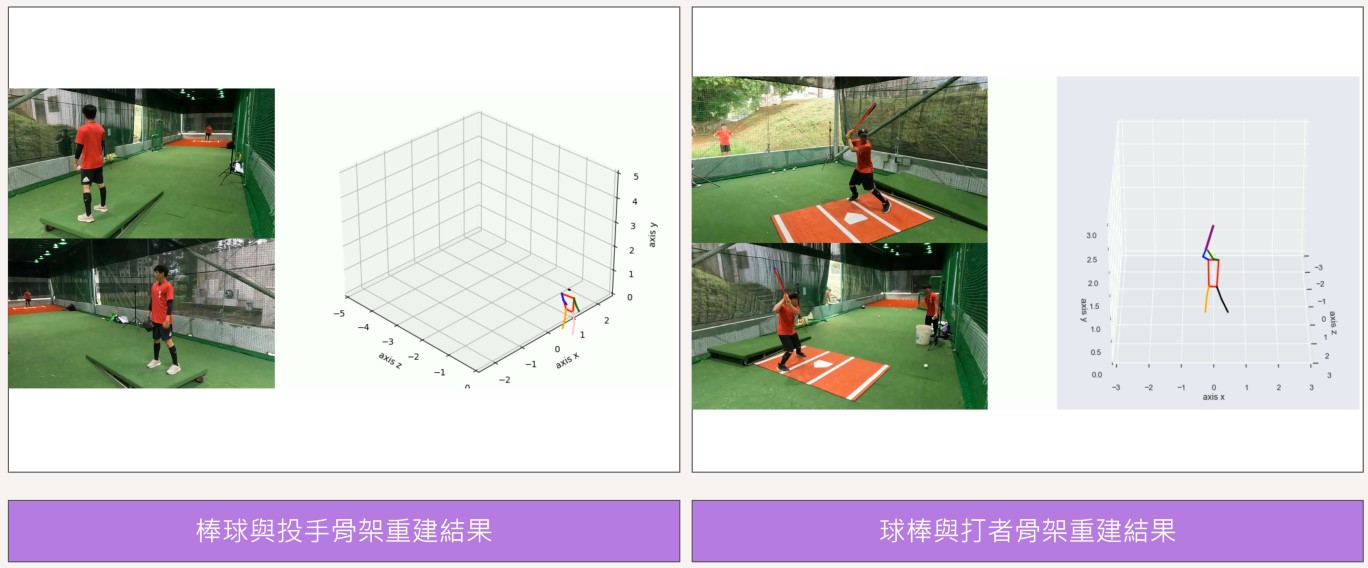
In advancing the development of the intelligent boxing arena, we've collaborated closely with national boxer Nien-chin Chen 's team. Together, we've installed a camera array to meticulously capture boxer movements for detailed analysis. Our focus includes developing algorithms for posture estimation, movement analysis, and real-time generation of movement instructions. These innovations enable us to provide immediate feedback and interactive coaching to enhance movement corrections effectively.
Traditional boxing training systems often struggle to replicate complex strike combinations and footwork. To address this limitation, we've introduced the MovableBag+ , a novel robotic system capable of dynamic movement and resistance.
The existing boxing training systems cannot provide complex combinations of punches and footwork. Moreover, commercial moving punching bags need a track fixed to indoor walls or beams. To address this, MovableBag+, a mobile and impact-resistant mixed reality robot was developed. MovableBag+ uses encountered-type haptic feedback technology to allow boxers to train their punches and footwork, reducing the burden on trainers. This technology provides tactile feedback resistance on physical objects, simulating obstacles in virtual reality. Integrated with VR visual feedback, MovableBag+ can simulate opponent appearances, transforming into a versatile mobile boxing training system with immersive multi-sensory feedback. Demonstrated both domestically and internationally over the past year, MovableBag+ has garnered acclaim for its innovative approach to boxing training (Figure B-21).
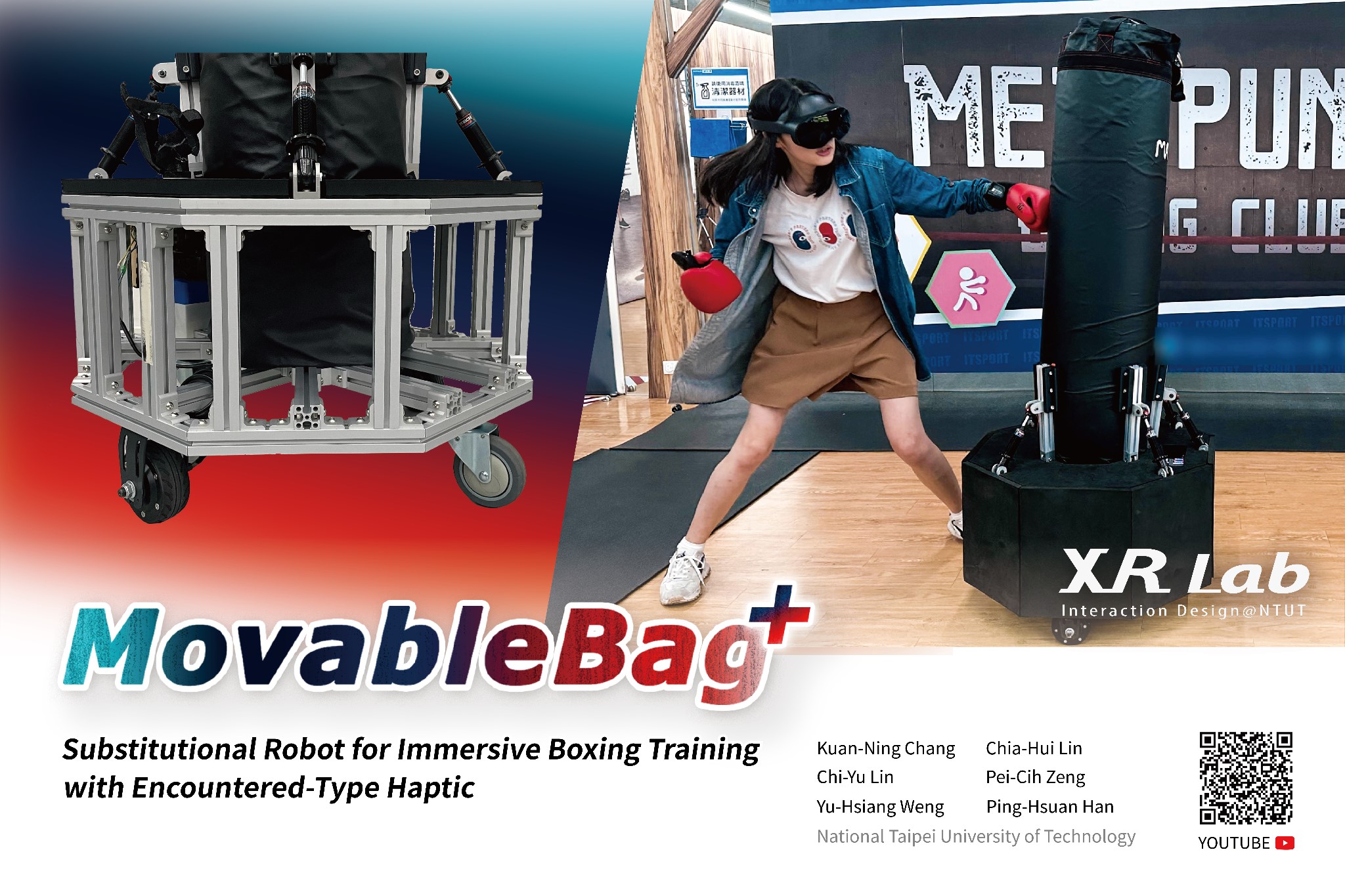
Fig. B-21, MovableBag+, an alternative reality robot that combines tactile feedback technology for immersive boxing training.
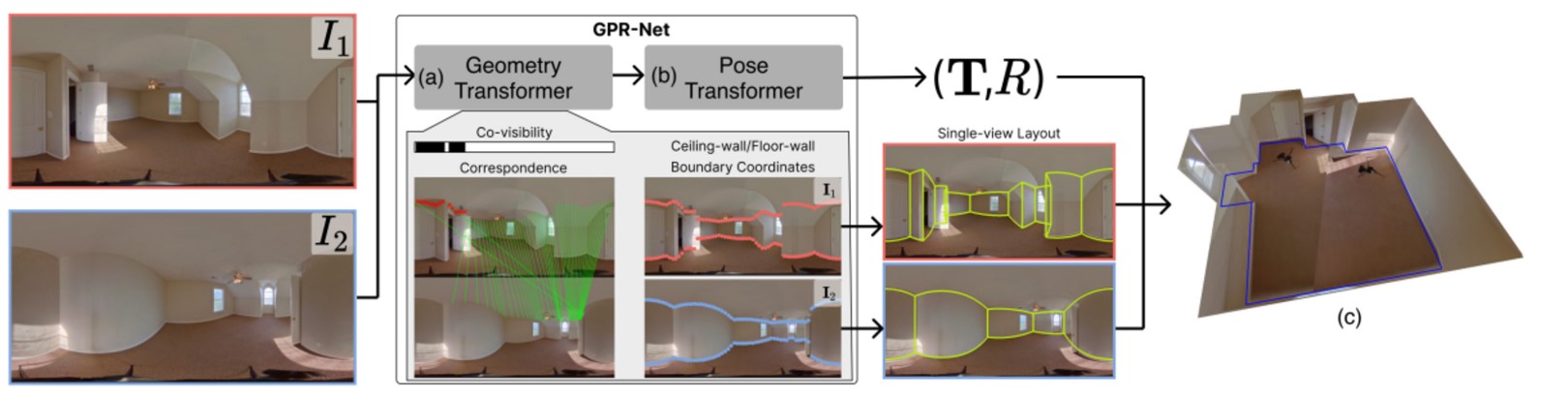
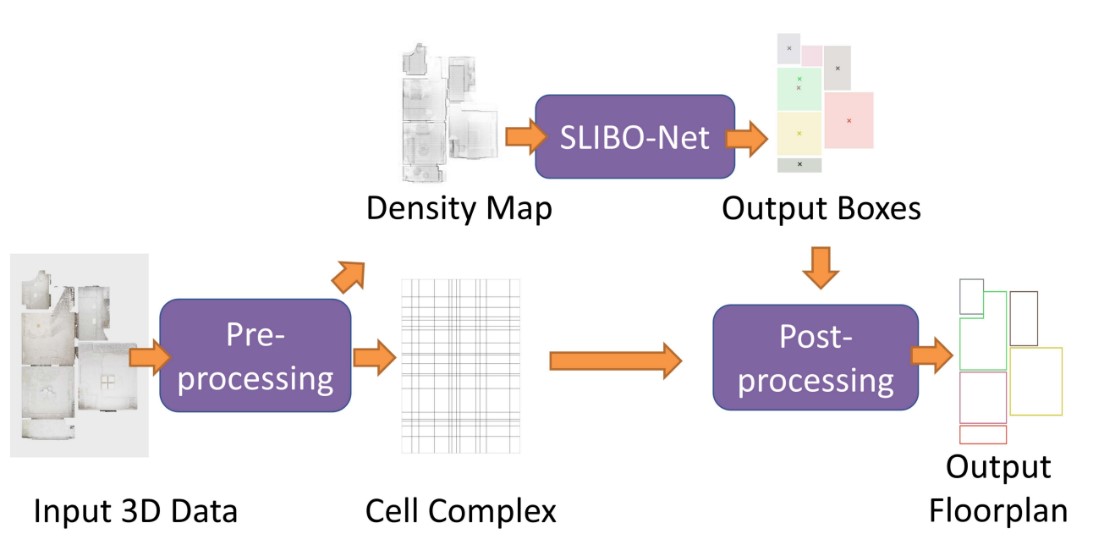
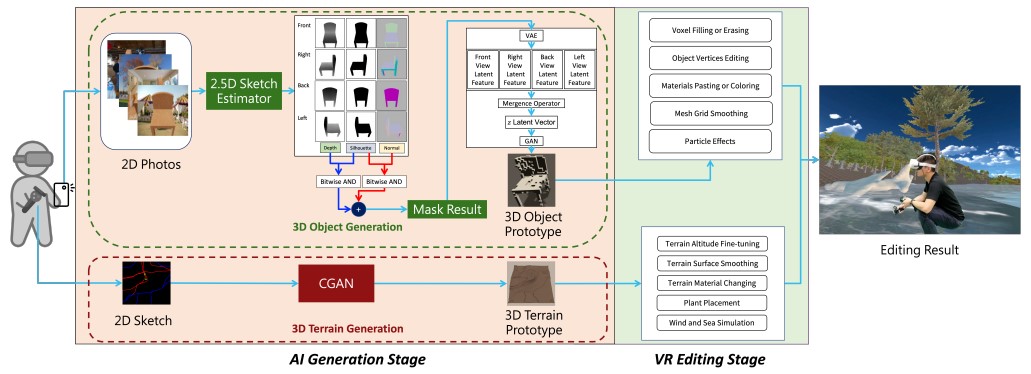
In addition, we have explored the recent mainstream Neural Radiance Fields (NeRF), Diffusion Model, and Gaussian Splatting methods for 3D content generation, and developed an algorithm for generating 3D point clouds of objects in a few-shot manner. We also adopted the popular GPT framework to construct the semantic relationship between text and motion sequences, and initially realized text-to-motion and motion-to-text generation. In this year, we have also completed the image-based 3D face reconstruction and expression tracking technology, which can reconstruct the full-face expression image of a VR user when his/her face is covered by a helmet, and the related results were presented at the ACM International Conference on Multimedia Retrieval (ICMR).
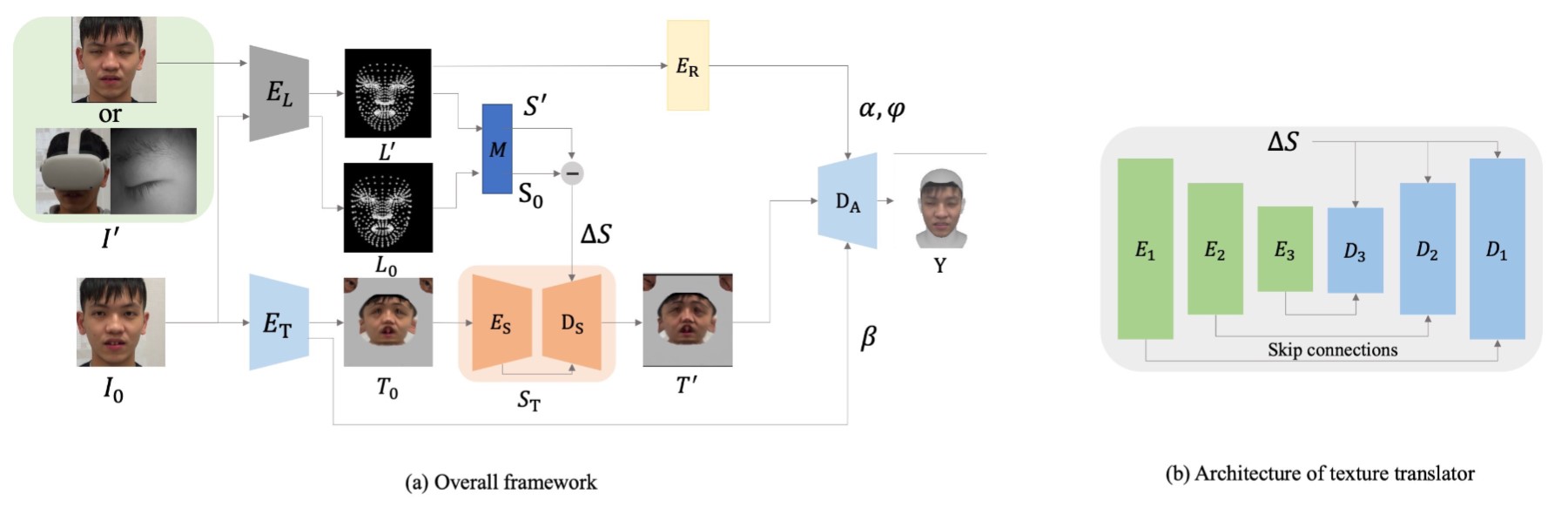
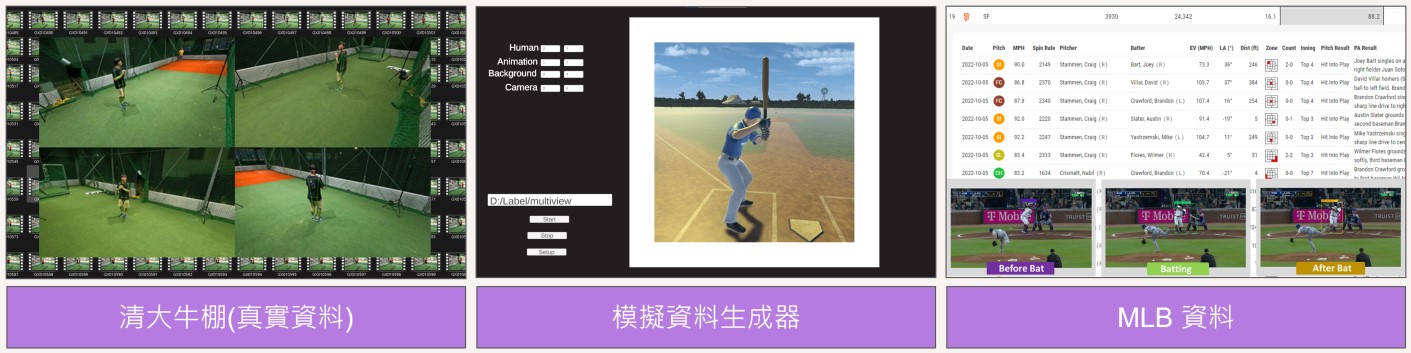
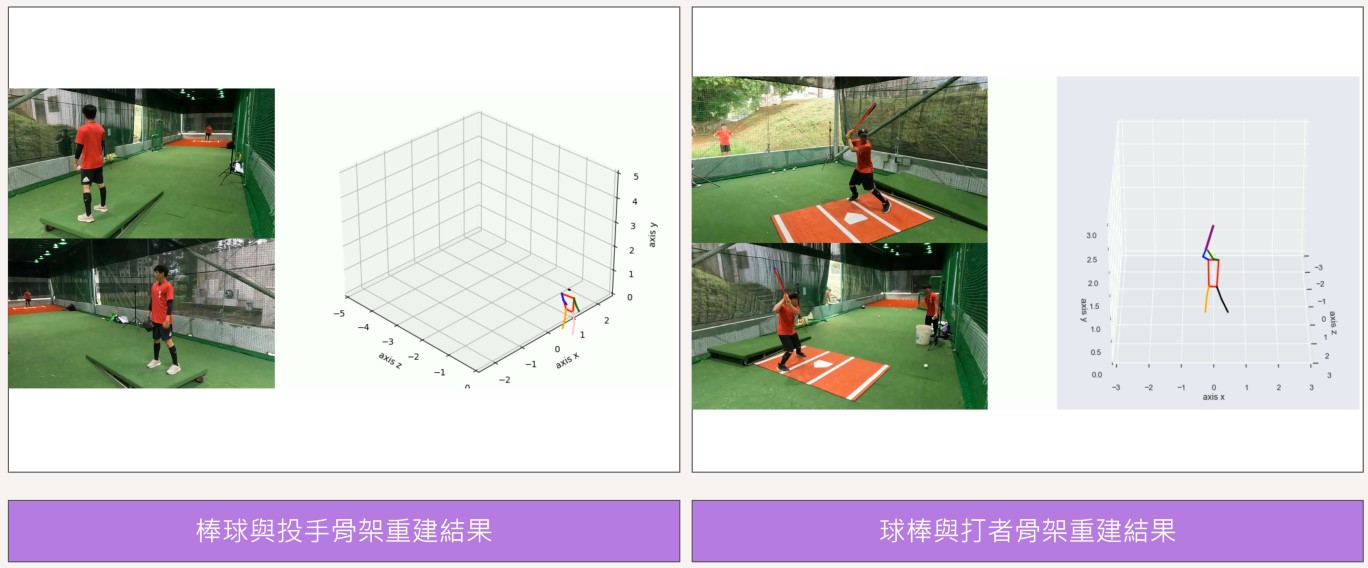
In advancing the development of the intelligent boxing arena, we've collaborated closely with national boxer Nien-chin Chen 's team. Together, we've installed a camera array to meticulously capture boxer movements for detailed analysis. Our focus includes developing algorithms for posture estimation, movement analysis, and real-time generation of movement instructions. These innovations enable us to provide immediate feedback and interactive coaching to enhance movement corrections effectively.
Traditional boxing training systems often struggle to replicate complex strike combinations and footwork. To address this limitation, we've introduced the MovableBag+ , a novel robotic system capable of dynamic movement and resistance.
The existing boxing training systems cannot provide complex combinations of punches and footwork. Moreover, commercial moving punching bags need a track fixed to indoor walls or beams. To address this, MovableBag+, a mobile and impact-resistant mixed reality robot was developed. MovableBag+ uses encountered-type haptic feedback technology to allow boxers to train their punches and footwork, reducing the burden on trainers. This technology provides tactile feedback resistance on physical objects, simulating obstacles in virtual reality. Integrated with VR visual feedback, MovableBag+ can simulate opponent appearances, transforming into a versatile mobile boxing training system with immersive multi-sensory feedback. Demonstrated both domestically and internationally over the past year, MovableBag+ has garnered acclaim for its innovative approach to boxing training (Figure B-21).
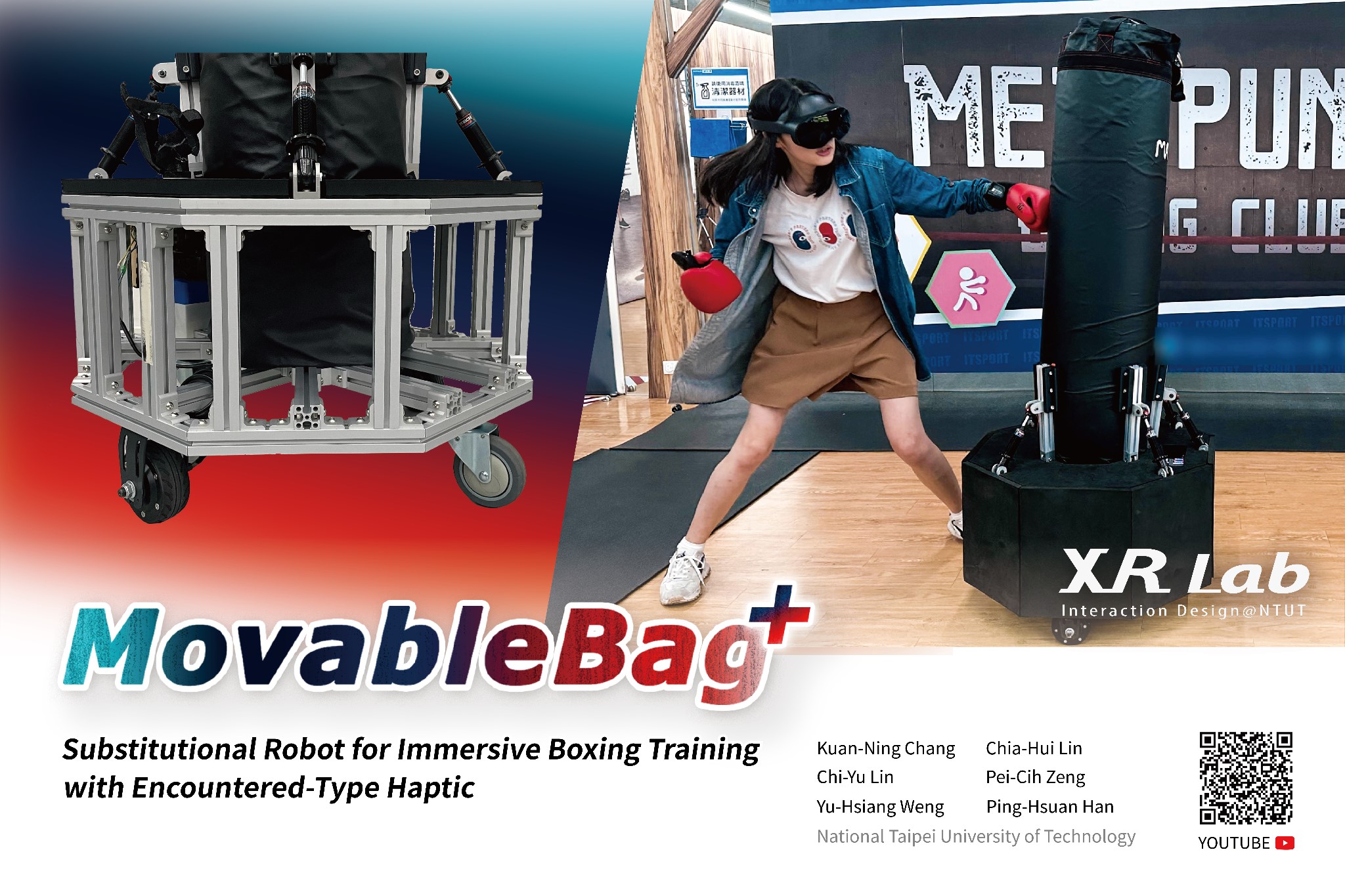
Fig. B-21, MovableBag+, an alternative reality robot that combines tactile feedback technology for immersive boxing training.
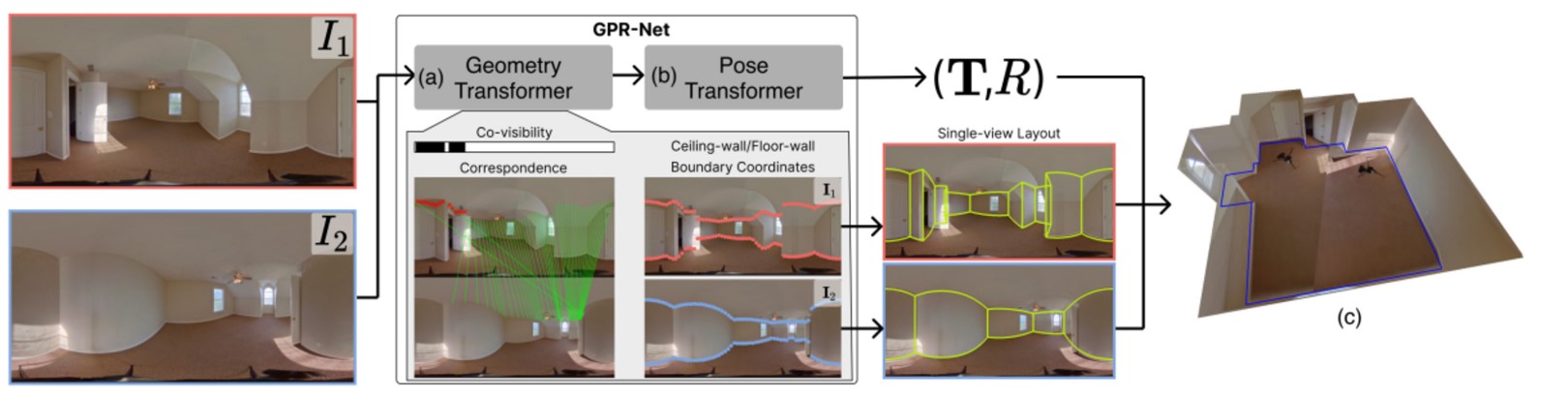
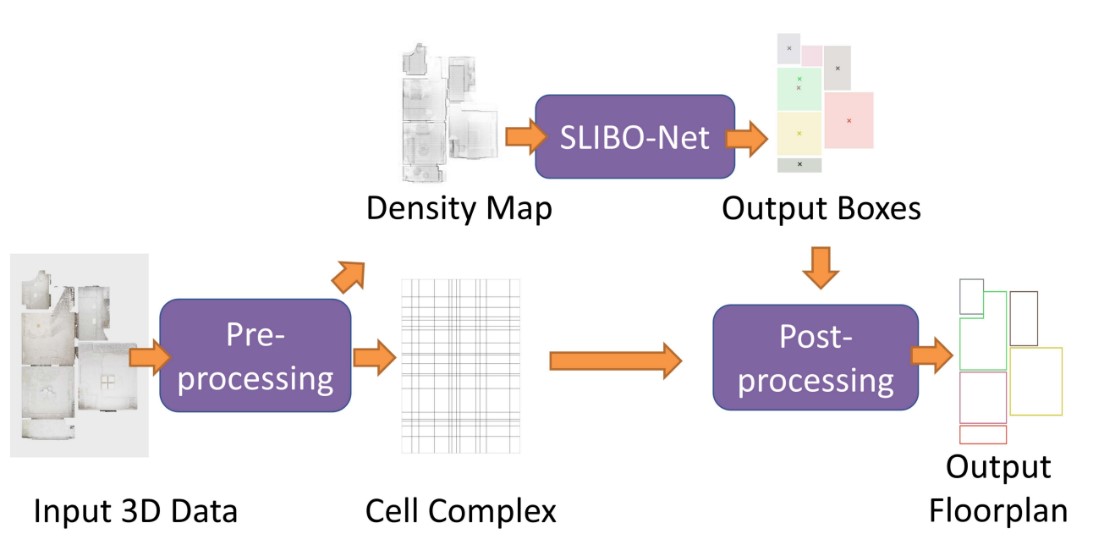
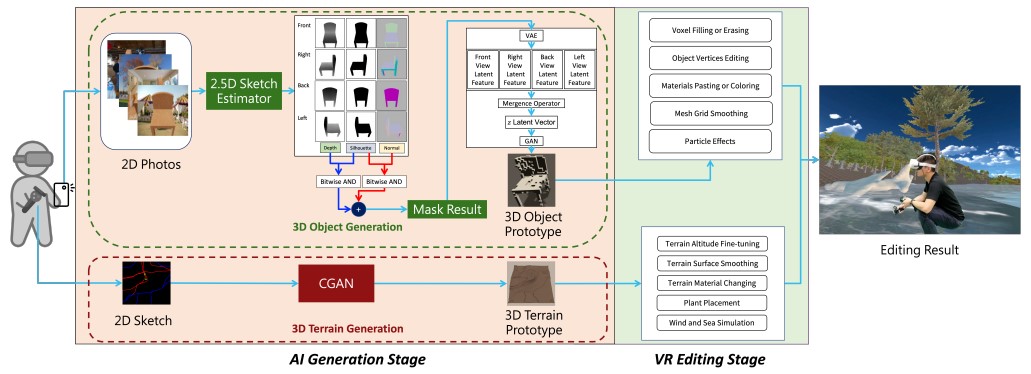
In addition, we have explored the recent mainstream Neural Radiance Fields (NeRF), Diffusion Model, and Gaussian Splatting methods for 3D content generation, and developed an algorithm for generating 3D point clouds of objects in a few-shot manner. We also adopted the popular GPT framework to construct the semantic relationship between text and motion sequences, and initially realized text-to-motion and motion-to-text generation. In this year, we have also completed the image-based 3D face reconstruction and expression tracking technology, which can reconstruct the full-face expression image of a VR user when his/her face is covered by a helmet, and the related results were presented at the ACM International Conference on Multimedia Retrieval (ICMR).
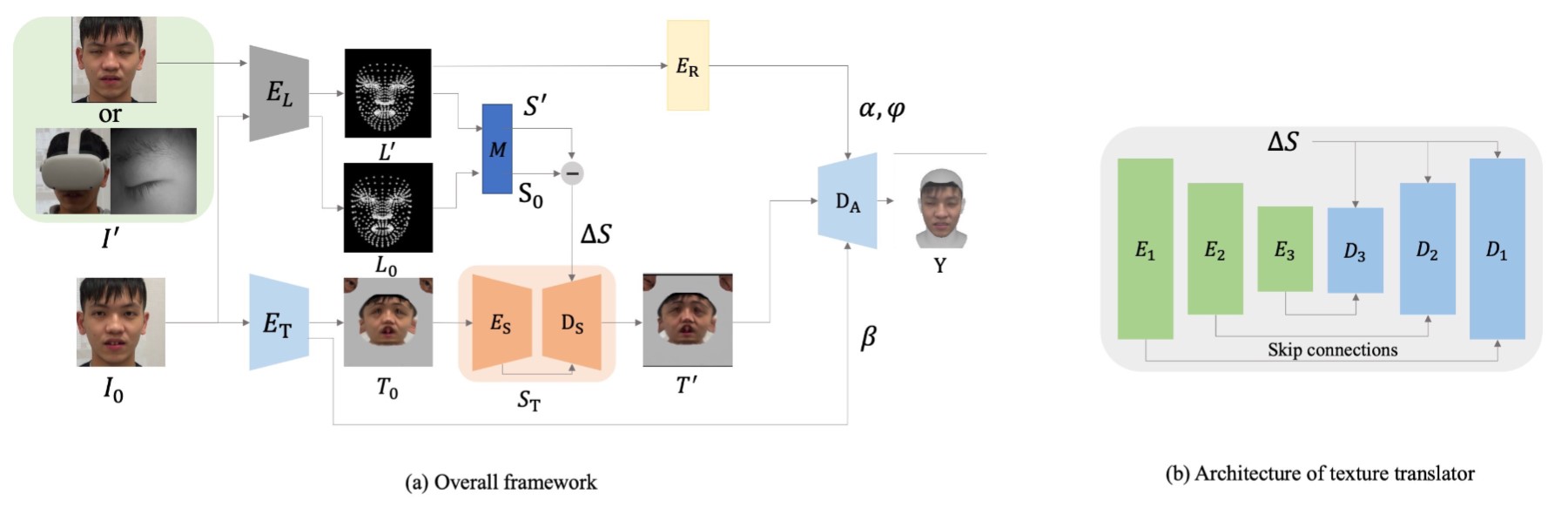